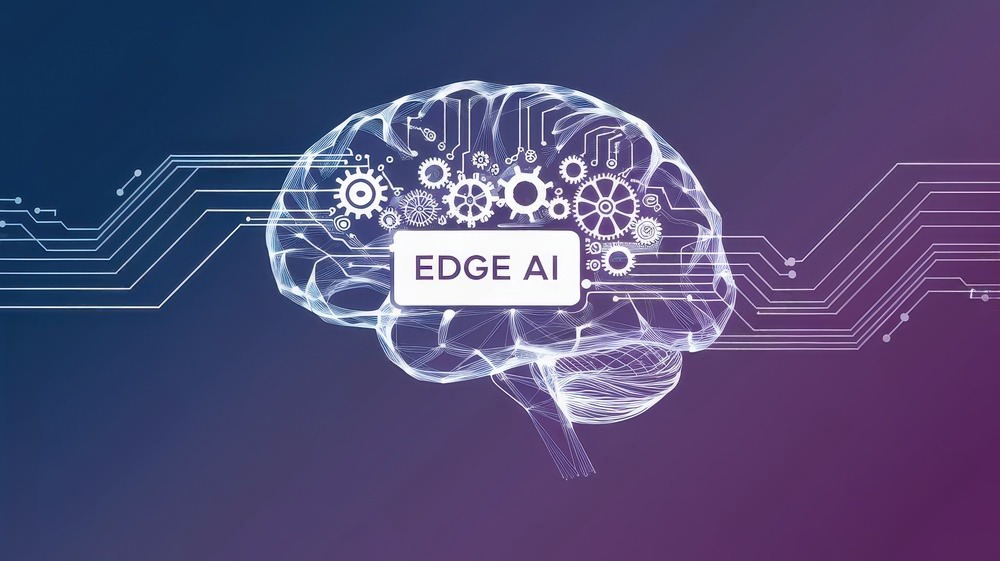
In today’s fast-paced tech era, edge machine learning is making a huge impact. It brings artificial intelligence closer to devices. As a result, gadgets work faster and more efficiently. Moreover, this approach often boosts privacy and cuts costs. In this post, we will explore how edge ML works, its benefits, real-world applications, challenges, and future opportunities.
1. Understanding Edge Machine Learning
Edge machine learning means running AI algorithms directly on local devices. Instead of sending data to a faraway cloud, a device processes it on the spot. Consequently, the response times are much lower. In addition, it minimizes data transfer costs.
1.1 What Does It Mean?
- Local Processing: Data is processed on your device.
- Faster Reactions: Results are available in near real time.
- Less Dependency: Reduced reliance on cloud servers.
For instance, think about a smart camera that can detect motion without internet help. To learn more, visit TechCrunch’s coverage on edge AI.
1.2 Training Versus Inference
There are two stages in machine learning. First, training builds a model on powerful servers. Second, inference uses that model for predictions. With edge ML, the inference happens right on the device. This makes everyday operations smoother and faster.
2. Major Benefits of Edge Machine Learning
Edge ML offers many advantages. Let’s discuss these benefits one by one.
2.1 Improved Speed and Real-Time Decisions
Because data is processed locally, response times are extremely short. Consequently, applications like autonomous vehicles and industrial robots can make split-second decisions. This low latency is essential for safety and efficiency.
2.2 Enhanced Privacy and Security
When sensitive data is handled on the device itself, the risk of interception is reduced. In addition, local processing lowers the chance of data breaches during transmission. This is particularly important for healthcare and finance sectors.
2.3 Reduced Bandwidth Costs
By processing data at the edge, less data travels over the network. As a result, bandwidth usage drops significantly. This reduction not only cuts costs but also eases network congestion.
2.4 Reliable Offline Functionality
Edge ML lets devices work without a continuous internet connection. For example, smart home devices and wearables can function locally. Consequently, they continue operating even if connectivity is lost.
2.5 Lower Cloud Dependency
Offloading tasks to local devices reduces the load on cloud servers. This helps cut cloud computing expenses over time. In addition, businesses become less vulnerable to cloud outages.
3. Edge Machine Learning in Real-World Applications
Edge machine learning is not just a concept—it is transforming various industries. Below are some practical examples.
3.1 Autonomous Vehicles
Self-driving cars rely on edge ML to process real-time sensor data. For instance:
- Object Detection: The car identifies obstacles immediately.
- Predictive Maintenance: Systems monitor components and alert for repairs.
- Dynamic Routing: Vehicles adjust routes as traffic conditions change.
These capabilities enhance both safety and reliability.
3.2 Smart Home Devices
Smart homes use edge ML to power many functions:
- Voice Assistants: They respond quickly to commands.
- Security Cameras: They can detect unusual activity without delay.
- Energy Management: Thermostats learn user habits to optimize temperature.
Local processing ensures these devices are more responsive and private.
3.3 Industrial IoT (IIoT)
In factories and warehouses, edge ML is used for:
- Predictive Maintenance: Sensors detect issues before machines fail.
- Quality Control: Real-time monitoring helps catch defects early.
- Automation: Robots and sensors work together to streamline operations.
This leads to smoother processes and lower downtime.
3.4 Healthcare Innovations
Edge ML is transforming medical care:
- Wearable Devices: They monitor vital signs and alert users instantly.
- Mobile Imaging: Devices can analyze scans right on site.
- Remote Monitoring: Patients in rural areas benefit from real-time analysis.
For more on these technologies, check Forbes’ tech insights.
4. Challenges and Opportunities
Even though the advantages are clear, there are challenges to overcome.
4.1 Limitations of Edge Devices
Edge devices usually have limited processing power compared to cloud servers. Therefore, models must be optimized to run efficiently on small hardware. In addition, memory and storage limitations can affect performance.
4.2 Security Concerns
Managing many dispersed edge devices poses unique security risks. Each device must be secured to protect data. This requires robust management tools and protocols.
4.3 Integration Complexities
Integrating edge ML with legacy systems can be challenging. Companies need to ensure compatibility between new models and old infrastructures. However, these challenges also create opportunities for innovation and improved solutions.
4.4 Scalability Prospects
Despite these hurdles, the field is growing. New platforms are emerging to manage edge AI workloads more effectively. Partnerships between technology providers and startups are accelerating progress. As a result, scalability and efficiency are improving continuously.
5. The Future of Edge Machine Learning
The impact of edge ML is expected to increase dramatically. Here are a few trends to watch:
5.1 Advances in Hardware
New chip designs and efficient processors will enable even more powerful edge devices. Consequently, more complex models can run locally.
5.2 Enhanced Software Optimization
Researchers are developing better techniques to compress and optimize models. This means you will see more robust AI on smaller devices.
5.3 Expanding Industry Adoption
From healthcare to smart cities, more sectors will integrate edge ML. This widespread adoption will transform everyday interactions with technology.
For recent updates on hardware trends, visit The Next Web.
6. Practical Tips for Startups and Developers
If you are a startup or developer looking to implement edge ML, consider the following:
- Start Small: Begin with a pilot project on one device.
- Optimize Your Models: Use quantization and pruning to reduce model size.
- Monitor Performance: Continuously test and update your devices.
- Collaborate: Join communities and forums to share best practices.
These tips help you get started without overwhelming resources.
Conclusion: Embracing a Smarter Future
To sum up, edge machine learning is a game changer. It brings powerful, real-time AI processing to local devices. In turn, this leads to faster decisions, enhanced privacy, lower costs, and improved reliability. Despite some challenges, the opportunities for innovation are immense. As hardware improves and integration becomes smoother, edge ML will only grow in importance.
We hope this comprehensive guide has given you a clear understanding of edge ML and its impacts. Please share your thoughts or questions in the comments below. We are excited to hear from you and help you explore this transformative technology further.